Hello Interactors,
I’ll admit it, the early summer weather here in Seattle has been a distraction. So, I turned to a writing companion this week to help. I took my notes from a talk I saw at the AAG conference in March, and you know what comes next…I pasted it in ChatGPT.
I then took some papers referenced in the talk, grabbed their abstracts, and pasted those in ChatGPT. After a few iterations of ‘expand on this’, ‘merge these…’ and ‘shrink this to three sentences’ I had all the working components needed to form an essay roughly 1000 words long.
I pasted that into Word and began editing in my own voice and adding bits of my own thoughts. When I’d get stuck on a concept or blending of concepts, I’d ask ChatGPT to blend them for me. When I was unsure or dubious of its confidence, I’d turn to Google Scholar and Wikipedia for further reference or content to be rewritten more evidentially.
I find ChatGPT a valuable writing companion. Some facts are often way off and downright fictional at times, but it is good at taking a jumble of ideas, lists of points, or disparate topics and putting them into cohesive starting points. Especially when the sun is shining and it’s 72 degrees outside.
Let me know what you think and what your thoughts are on using ChatGPT for this kind of thing. Here’s what ChatGPT says are three takeaways from this post.
Key Takeaways:
Grid and Place Cells: Researchers have discovered specific patterns of neuron firing in the brains of rats, mice, bats, and humans, known as place cells and grid cells. Place cells are found in the hippocampus and respond to specific locations in the environment, while grid cells in the entorhinal cortex form grid-like patterns representing the overall layout of space.
Non-Euclidean Geometries: The discovery of grid cells and cognitive maps challenges the dominance of Euclidean geometry in understanding spatial cognition. Grid cells exhibit a hexagonal grid pattern, highlighting the non-Euclidean nature of cognitive maps in the brain.
Computational Models: The integration of computational models such as reinforcement learning and successor representations, inspired by the research on place cells and grid cells, offers a deeper understanding of spatial cognition. These models capture the complex interactions between cognition, decision-making, and the environment, reflecting the non-Euclidean nature of grid cells and challenging traditional geometric frameworks. By incorporating these computational approaches, researchers can develop more comprehensive and inclusive models of spatial cognition that consider the interconnectedness and dynamic nature of cognitive processes and the environments we navigate.
As interactors, you’re special individuals self-selected to be a part of an evolutionary journey. You’re also members of an attentive community so I welcome your participation.
Please leave your comments below or email me directly.
Now let’s go…
TELLTALE CELLS OF RAT TRACES
In my last post, I talked about how Platonic and Euclidian geometric purity and gridded Cartesian planes may have overly influenced Western thought, beliefs, and actions. Scientific thinking may have been errantly seduced by the certainty and accuracy of rational proofs to describe not only the physical world but how our minds and body interact with it and each other. We now know it’s likely the world is constructed of layers of indeterminant, interrelated complex interactions occurring at atomic, chemical, biological, psychological, sociological, astronomical, and cosmological levels.
And yet theoretical and observable patterns emerge amidst this chaos that can and are described and understood through both Euclidean and non-Euclidean patterns. For example, patterns found in the brain. Cognitive scientists studying rats in the 1970s discovered a collection of neurons fired when a rat entered a particular place in a maze. They called these neuron firing locations place cells.
This video shows a rat running around in a circular environment (black line) and any time a particular cell is active (red dots). The red dots cluster around one location, which is the place field of the cell. (Source: Jankowski M, O’Mara S (2015) via Wikipedia)
Place cell patterns are found primarily in the hippocampus region of the brain. They’re derived from a collection of firing neurons that selectively respond to specific locations or places in an environment. Place cells are influenced by environmental cues and play a crucial role in spatial memory formation, allowing for the association of specific memories or experiences with particular places. They are instrumental in the formation of cognitive maps, which are inherently non-Euclidean because they can include unpredictable shortcuts, deviations, and distortions.
As research continued in the 1990s, the arrangement of these patterns began showing signs of grid-like regularity. Some speculated the brain was forming a kind of rectangular grid. And then in 2005, scientists discovered grid-like patterns firing in an area neighboring the hippocampus, the entorhinal cortex – also involved in spatial processing and memory. They termed these grid cells and they indeed resembled Euclidean regularity.
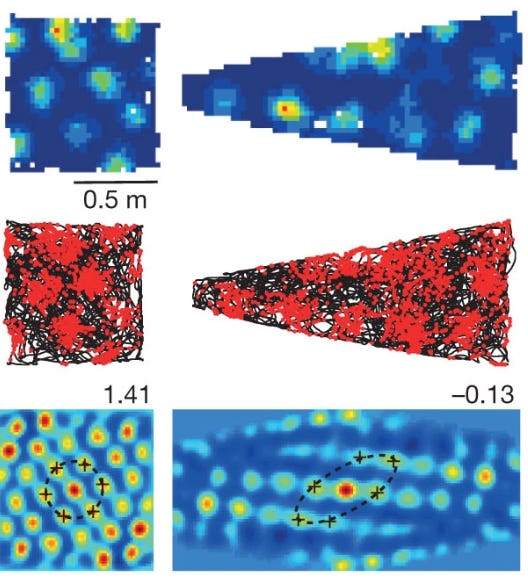
These collections of neurons fire at regular intervals as a rat moves about the environment. As it nears a node of its virtual lattice, neurons fire in the entorhinal cortex. These nodes make a grid-like pattern, but it’s not a regular rectangular grid. It instead is comprised of hexagonal shapes. Unlike place cells, which have specific firing fields for distinct locations, grid cells offer a continuous representation of space, capturing the overall layout and virtual hexagonal geometry of the environment in the brain.1
By establishing a kind of coordinate system, scientists theorize grid cells are used to monitor movement and position relative to the surrounding space. This supports path integration and reinforcement which helps maintain a sense of direction and distance traveled. While the eyes play a crucial role in strengthening these patterns, the lattice is nonetheless present even when traversing in the dark – absent of external cues.
While this phenomenon has been found in the brains of rats, mice, bats, and humans, similar findings exist in insects, albeit sparse and more static. Neurons in their antennal lobe encode the identity of odors through patterns formed by synchronized oscillating feedback loops with other neurons responsible for olfactory processing and learning.
Research on place cells, grid cells, and other brain structures involved in spatial representation and learning can provide valuable insights into how humans perceive and navigate space. By understanding the neural mechanisms underlying spatial cognition, researchers can refine and validate cognitive models of space.
MOST CERTAINLY A CERTAIN UNCERTAINTY
While I was attending the American Association of Geographer’s conference in Denver last March, I attended a session aimed at a body of research called behavioral and cognitive geography (BCG). This discipline has opened new avenues for understanding the links between human cognition, behavior, and the environment. This interdisciplinary field combines concepts from geography, psychology, neuroscience, AI, and other disciplines to explore how individuals and groups interact with space and make decisions individually and collectively.
One important aspect of BCG is decision making. Researchers in this field aim to develop more representative models of decision making by incorporating insights from disciplines such as judgment and decision making. This involves moving away from unrealistic assumptions grounded in traditional, rational interpretations in geography using Euclidean geometry. Two of my professors at UCSB, Reg Golledge2 and Waldo Tobler3, both called for non-Euclidean investigations after forays into their groundbreaking research in cognitive maps in the 1970s.
Advancing this knowledge will require accounting for the context of spatial decision making. This includes social norms, institutions, and the physical environment that influences us. Additionally, acknowledging the heterogeneity among decision makers and considering cultural values is crucial for developing comprehensive decision models that are representative of all groups.
Collaborations with fields such as artificial intelligence, computational neuroscience, and social physics are contributing to expanding the scope and ambition of BCG. One researcher in this area from the University of Leeds, Ed Manley, presented at the conference. He is developing new analytical methods that blend topological and computational neuroscience, like those found in grid and place cell research, to account for both Euclidean and non-Euclidean geometries and explanations.
His recent research integrates both cognitive and behavioral geography using advanced agent-based modeling and spatial analysis. By leveraging the availability of large-scale, rich data on human mobility with computational models of spatial cognition he sees opportunities for deepening our understanding of how people interact with place.
There are two computer-based models he is currently exploring – reinforcement learning (RL) and successor representations (SR). These concepts are influenced and inspired by advancements in neuroscience – including place cell and grid cell research. Reinforcement learning is a general framework for learning optimal behavior through interactions with an environment while successor representations are a specific method used within reinforcement learning.
Reinforcement learning involves an agent that learns to make decisions and take actions in an environment to maximize cumulative rewards. The agent interacts with the environment, receives feedback in the form of rewards or penalties, and adjusts its behavior based on this feedback. The goal is to learn rules that map states to actions to maximize the expected cumulative reward.
Successor representations represent the state transitions and expected future rewards associated with different states. Instead of directly representing states and their values, successor representations capture the underlying structure of the environment. Probability scores are mapped to transitions between states and the expected cumulative reward that can be obtained from each state.
The modeled probability values of a state relates to the expected cumulative reward obtained by starting from that state and following a particular set of rules. By representing states in terms of their expected future rewards, successor representations provide a more compact and informative representation of the environment.
COMPLEXITY AND CONNECTION IN SUCCESSOR EVOLUTION
In the context of reinforcement learning, successor representations can be used to facilitate learning and decision-making by providing a structured representation of the environment's dynamics. This allows computer-based agents to make better-informed decisions based on the expected future rewards associated with different states.
Manley’s integration of SR into cognitive geography holds promise for understanding the construction of spatial knowledge and its connection to cognition. This method captures the idea that previous choices inform future decisions, mirroring the cognitive processes involved in spatial learning. This approach is closely linked to the spatial-dependent nature of tasks, which arise from geographic processes – including the formation of the natural and built environment.
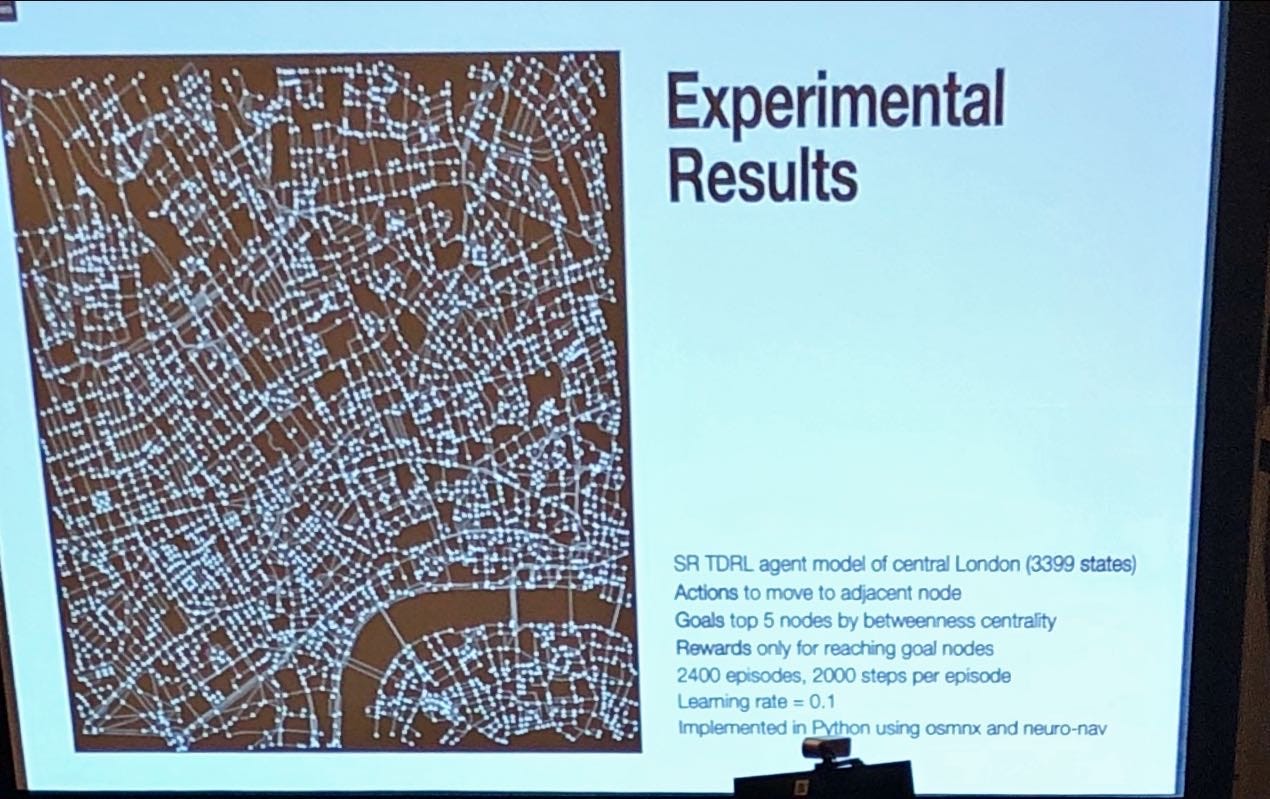
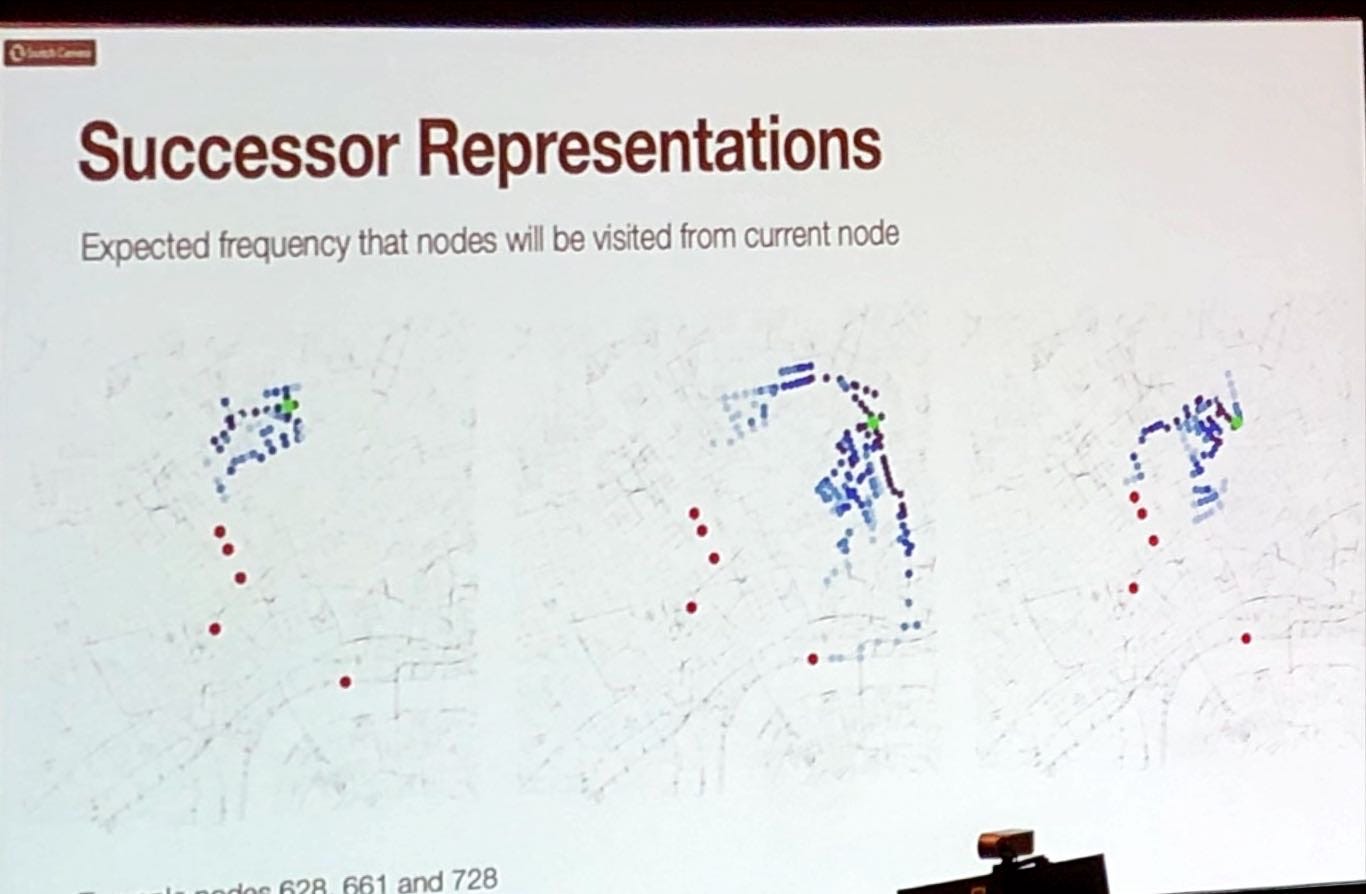
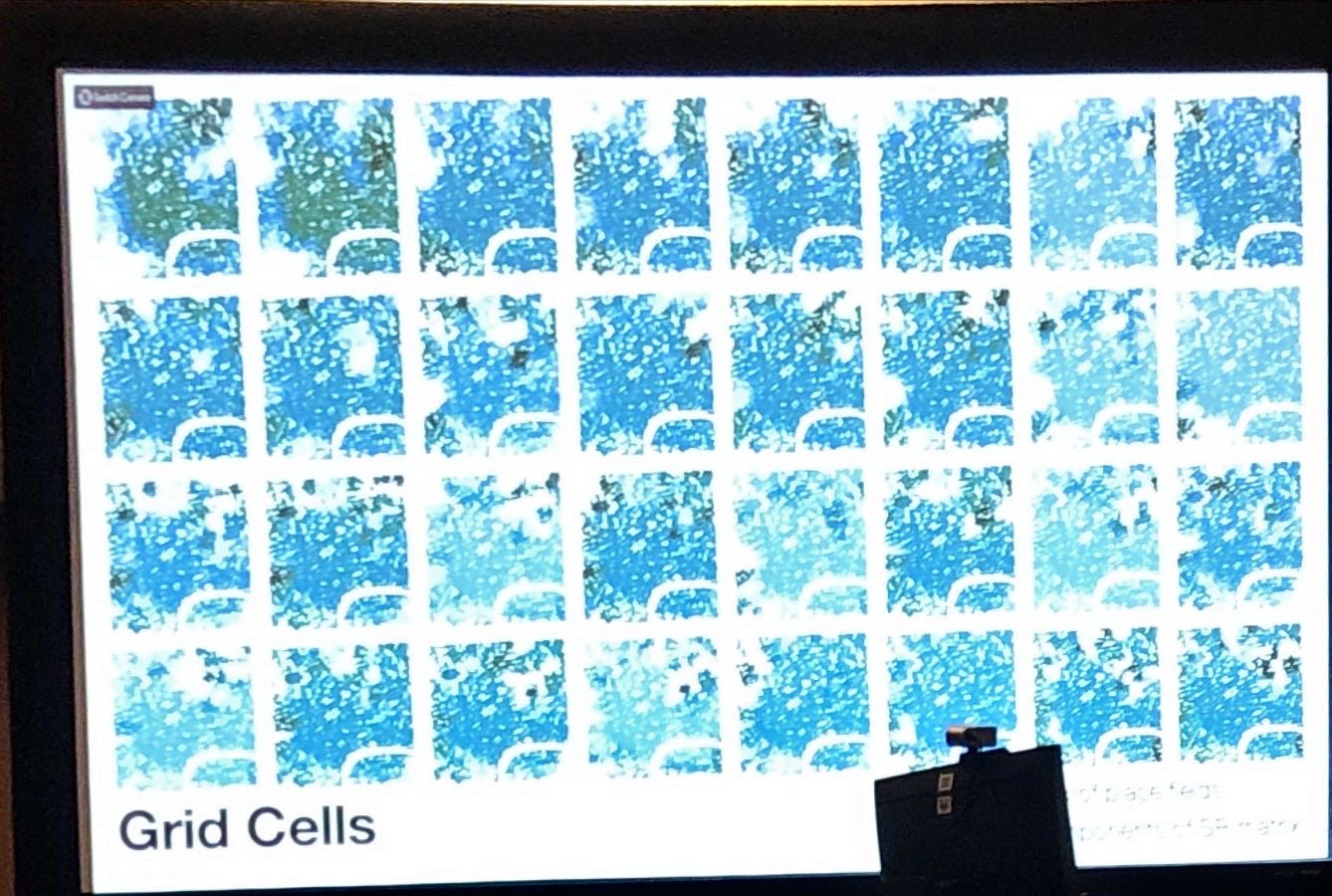
This research also shows potential for tailoring learning processes to known demographic and background factors, allowing for more fair, accurate, and representative models of spatial cognition. This enables researchers to account for the diverse influences that shape decision-making and spatial behavior among different populations and their environments.
Moreover, the utilization of these methods in cognitive geography provides an avenue to develop models of space that blend metric and topological representations systematically. This integration allows for a more comprehensive understanding of spatial environments, capturing both the quantitative measurements and the qualitative relationships between locations.
This approach to geography represents a departure from dominant Platonic, Euclidean, and Cartesian thinking, embracing complexity and connectedness. These computational approaches reflect and acknowledge the dynamic nature of cognition, where the iteration of past choices inform future decisions.
It highlights the interconnectedness between cognition and the environment, challenging compartmentalized views and fostering interdisciplinary approaches. This departure from fixed and predetermined paths echoes a broader shift towards embracing complexity in our understanding of the world. It encourages us to view spatial cognition as a dynamic process influenced by a multitude of factors, rather than relying solely on rigid geometric frameworks and dogma.
It invites us to move away from rigidity and instead embrace the dynamic, interwoven nature of our cognitive processes and the environments we navigate. By doing so, we can foster a more nuanced, inclusive, and holistic understanding of spatial cognition and representation that aligns with the intricacies and interdependencies of the world we inhabit.
Grid cell symmetry is shaped by environmental geometry. Julija Krupic, et al. Nature. 2015.
Golledge, R. G. and Spector, A. N. (1978). Comprehending the urban environment: theory and practice. https://doi.org/10.1111/j.1538-4632.1978.tb00667.x
Tobler, W. R. (1976). The geometry of mental maps. In Spatial Choice and Spatial Behavior (ed. R. G. Golledge and G. Rushton).
Share this post